Summary
Crafting realistic materials is essential in three-dimensional (3D) content creation, as it significantly enhances visual realism and the illusion of immersion. Traditionally, this task has involved extensive manual labor, but recent advancements in material reconstruction from photographs offer a promising alternative. These methods, which are often data-driven and utilize deep neural networks, have revolutionized the process through automation and simplification. Despite these advancements, most techniques still depend on controlled lighting conditions. In addition, it remains a substantial challenge to create materials from photographs taken in varied real-world environments, which is crucial to making further advancements in realistic and versatile 3D material creations. This research introduces novel methods for both material estimation and synthesis, catering to various aspects of photorealistic rendering. DiffMat combines the contrastive language-image pre-training (CLIP) image encoder with a multi-layer, cross-attention denoising backbone, enabling the generation of latent materials from images under a wide range of illuminations. DiffMat are designed for smooth integration into standard physically based rendering pipelines. Compared with state-of-the-art approaches, DiffMat produces material with higher quality, while also imposing fewer constraints on the reference images.
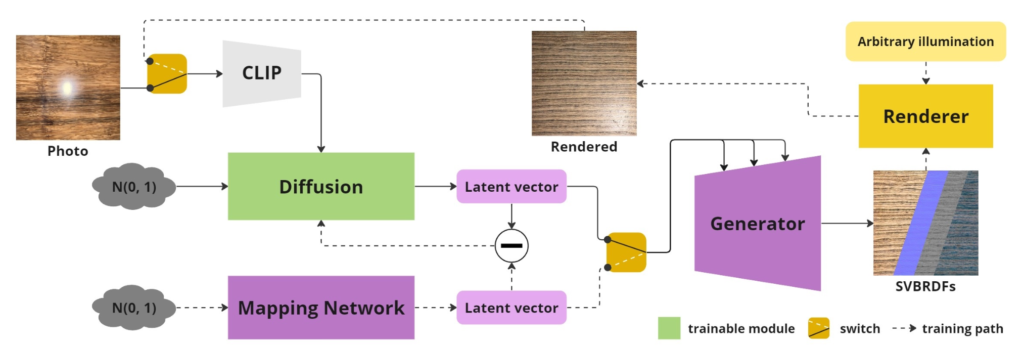
Members
Name | Affiliation | Web site |
---|---|---|
![]() |
Keio University | |
Dingkun Yan | Tokyo Institute of Technology | |
Suguru Saito | Tokyo Institute of Technology | https://www.img.cs.titech.ac.jp/en/ |
Publications
Journals
- Liang Yuan, Dingkun Yan, Suguru Saito, Issei Fujishiro: “DiffMat: Latent Diffusion Models for Image-Guided Material Generation,” Visual Informatics, Vol. 8, No. 1, pp.6–14, March 30, 2024 [doi: DOI].
Grants
Grant-in-Aid for Scientific Research (A): 21H04916 (2021)